The psychobabble behind the ‘AI is racist’ claim

Astonishing news is in. Apparently, artificial intelligence can be bigoted, too.
The claim comes from three Princeton IT scholars who made Global Vectors for Word Representation (GloVe), a popular algorithm that performs unsupervised learning from text, crawl through billions of words on the internet, assessing the meaning of particular words statistically by checking which other words were near them. They found that the GloVe algorithm made the same iffy associations between words that human beings do. Or, as Wired put it: ‘Just like humans, artificial intelligence can be sexist and racist.’
GloVe linked words such as ‘woman’ or ‘girl’ with the arts, rather than with mathematics. It linked African-American names with unpleasant words. From this, the IT scholars concluded that ‘machine learning absorbs stereotyped biases as easily as any other’. IT that can learn, understand and produce language, they wrote, will acquire ‘cultural associations, some of which can be objectionable’.
For them, it’s a worry that companies may screen job applicants using software that has come to ‘imbibe cultural stereotypes’. But this denudes sexism and racism of their real meaning. For them, these phenomena have no ‘explicit’ roots in the structures, economy, policy or institutions of the US – in labour utilisation or immigration control, for example. ‘Before providing an explicit or institutional explanation’ for why individuals make ‘prejudiced decisions’, the authors intone, ‘one must show that it was not a simple outcome of unthinking reproduction of statistical regularities absorbed with language. Similarly, before positing complex models for how stereotyped attitudes perpetuate… we must check whether simply learning language is sufficient to explain (some of) the observed transmission of prejudice.’
In other words, these experts believe sexism and racism are simply a result of unconscious – ‘implicit’ – prejudice. This is a common view, which underpins anti-bias training in universities and workplaces. But it is highly problematic: it trivialises oppression where it does still exist. From this perspective, progress lies not in taking on social structures that hold certain people back, but in improving our language and continually ‘updating’ AI in line with this.
But the way in which implicit bias is measured in both humans and machines is highly questionable. The Implicit Association Test (IAT), currently used to measure a subject’s sexism, racism and much else besides, was born out of the work of Anthony Greenwald and Mahzarin Banaji. In 1995, they argued that attitudes and stereotypes were activated unconsciously and automatically. And in 1998, Greenwald and two of his colleagues at the University of Washington put it to the test. Using just 17 Korean-American students, they found that the group took longer to respond favourably to a Japanese name than to a Korean name. The IAT is, to put it plainly, incredibly reductionist.
The Princeton scholars used the IAT to measure the prejudice of AI. Indeed, they believe their results ‘add to the credence of the IAT by replicating its results in such a different setting’ – namely, that of clever bots.
Might biased AI be a problem in future? Perhaps. But biased psychobabble is a real danger in the present.
Fmr President of Kenya on Trump cutting off foreign aid:
“Why are you crying? It’s not your government, he has no reason to give you anything. This is a wakeup call to say what are we going to do to help ourselves?”
America first is good for the world.
Our entire Green Socialist establishment should be banged up under the ‘Online Safety’ laws, for spreading demonstrable lies (the ‘climate crisis’), causing non-trivial harm to the industrial working class, ordinary drivers, farmers, taxpayers etc, etc.
#Chagos? #Mauritius PM Navin Ramgoolam "is reported to want Starmer to pay £800m a year, plus ‘billions of pounds in #reparations’." (14 January) https://www.spiked-online.com/2025/01/14/the-chagos-islands-deal-is-an-embarrassment/
Now the Torygraph wakes up https://telegraph.co.uk/gift/1ff8abbb462cd609
Read @spikedonline - first with the news!
Articles grouped by Tag
Bookmarks
Innovators I like

Robert Furchgott – discovered that nitric oxide transmits signals within the human body
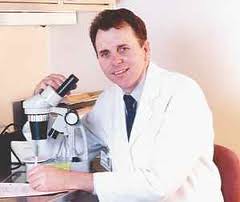
Barry Marshall – showed that the bacterium Helicobacter pylori is the cause of most peptic ulcers, reversing decades of medical doctrine holding that ulcers were caused by stress, spicy foods, and too much acid

N Joseph Woodland – co-inventor of the barcode
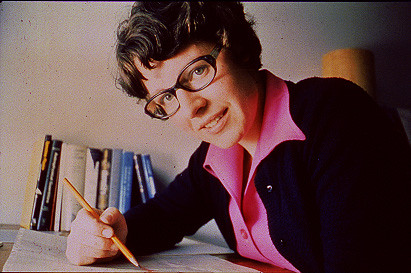
Jocelyn Bell Burnell – she discovered the first radio pulsars

John Tyndall – the man who worked out why the sky was blue

Rosalind Franklin co-discovered the structure of DNA, with Crick and Watson

Rosalyn Sussman Yallow – development of radioimmunoassay (RIA), a method of quantifying minute amounts of biological substances in the body

Jonas Salk – discovery and development of the first successful polio vaccine

John Waterlow – discovered that lack of body potassium causes altitude sickness. First experiment: on himself
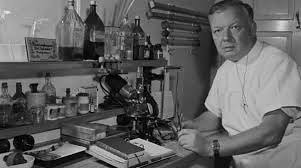
Werner Forssmann – the first man to insert a catheter into a human heart: his own

Bruce Bayer – scientist with Kodak whose invention of a colour filter array enabled digital imaging sensors to capture colour
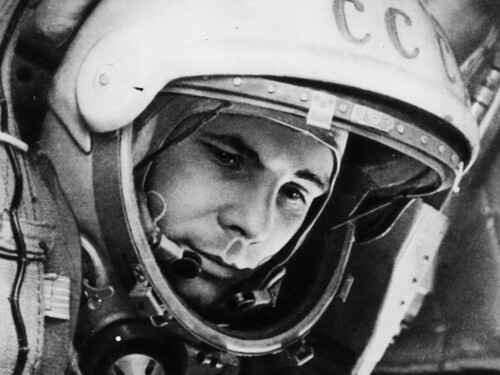
Yuri Gagarin – first man in space. My piece of fandom: http://www.spiked-online.com/newsite/article/10421

Sir Godfrey Hounsfield – inventor, with Robert Ledley, of the CAT scanner

Martin Cooper – inventor of the mobile phone

George Devol – 'father of robotics’ who helped to revolutionise carmaking

Thomas Tuohy – Windscale manager who doused the flames of the 1957 fire

Eugene Polley – TV remote controls
0 comments